In microbiome research (including metagenomics and 16S rRNA sequencing), the terms absolute abundance and relative abundance are frequently encountered. However, what exactly do these terms mean, and why is it important to differentiate between them?
What is Relative Abundance?
Relative abundance refers to the proportion of a specific microorganism within the entire microbial community. In other words, it does not provide the actual number of microorganisms but rather indicates the proportion of that microorganism relative to the total microbial count. The sum of relative abundances typically equals 100% (or 1).
Example:
Assume that in a sample, a total of 300,000 bacteria are detected, with 100,000 being species A and 200,000 being species B. The relative abundance can be calculated as follows:
- Relative abundance of species A = 100,000 / 300,000 = 33.33%
- Relative abundance of species B = 200,000 / 300,000 = 66.67%
Relative abundance is relatively straightforward to calculate, and since it is normalized to the total microbial count, it is unaffected by the total sample size. High-throughput sequencing techniques, such as 16S rRNA sequencing, are commonly used to obtain relative abundance data.
What is Absolute Abundance?
Absolute abundance refers to the actual number of a specific microorganism present in a sample. It is typically quantified as the "number of microbial cells per gram/milliliter of sample." This measure directly informs us about the actual quantity of microorganisms in the sample.
Example:
In a water sample, suppose 100,000 bacteria of species A and 200,000 bacteria of species B are detected. The absolute abundance of species A is 100,000 cells, and for species B, it is 200,000 cells.
Absolute abundance data is usually obtained through quantitative techniques such as quantitative PCR (qPCR), which require additional experimental steps and precise measurement tools.
Key Differences Between Absolute and Relative Abundance
The main differences between absolute and relative abundance are as follows:
- Absolute abundance provides the actual count of microorganisms, which reflects the true number of microbes in the sample.
- Relative abundance describes the proportional relationship between different microorganisms within a sample, allowing for comparison of their relative distributions.
However, a limitation of relative abundance is that it may not accurately reflect the true changes in a microorganism's abundance when the total sample size varies. For instance, if the numbers of both species A and species B decrease proportionally, the relative abundance might remain unchanged, even though the actual number of these microorganisms has decreased. In contrast, absolute abundance would reveal the actual decrease in microbial numbers.
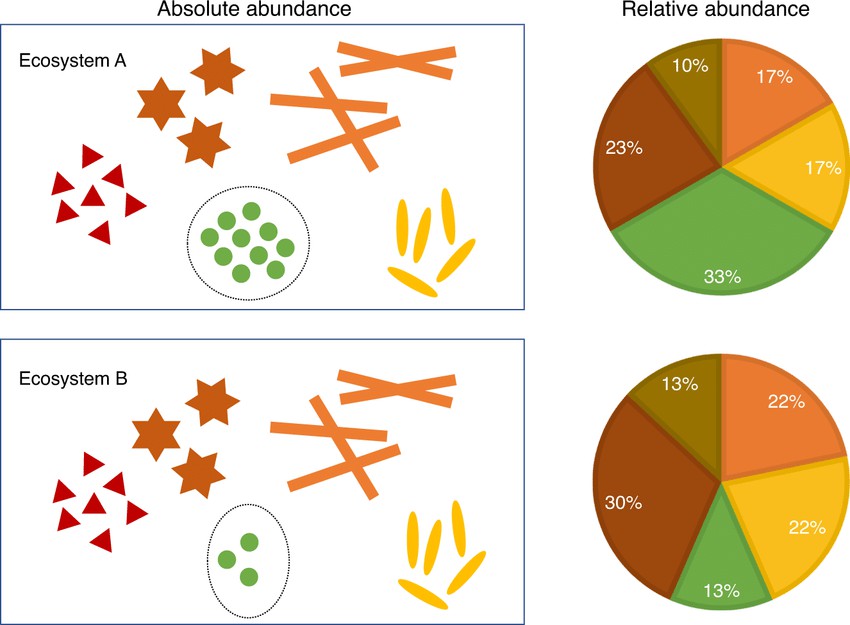
Figure 1. The distinction between absolute abundances and relative abundances (Huang Lin et al., 2020)
When to Use Absolute Abundance and When to Use Relative Abundance?
Absolute abundance: If the goal is to determine the actual number of microorganisms (such as in disease monitoring or precise quantification of microbial load), absolute abundance is more reliable.
Relative abundance: If the focus is on understanding the community structure and comparing the proportions of different microorganisms within a sample (such as in ecological studies of microbial populations), relative abundance is often preferred. This approach highlights the proportional relationships among microbes within the community.
By understanding these two different approaches, researchers can select the appropriate method for their specific study objectives, ensuring that the data obtained provides meaningful and accurate insights into the microbiome.
Absolute and Relative Abundance in 16S rRNA Sequencing
16S rRNA sequencing is a widely employed technique for analyzing microbial community structure. It works by amplifying the 16S rRNA gene of bacteria and archaea, which helps identify the types of microorganisms present in a sample.
In 16S sequencing, relative abundance is commonly used. This is because the sequencing results typically provide the sequence reads for each bacterial taxon (e.g., operational taxonomic units (OTUs) or amplicon sequence variants (ASVs)), rather than the actual quantity of organisms. Variations in sequencing depth and efficiency can influence the total number of sequences across different samples, prompting the conversion of sequence counts into relative abundance for comparison between samples.
To determine absolute abundance in 16S rRNA sequencing, additional methods, such as qPCR or flow cytometry, are required to quantify the total microbial load in the sample. The absolute abundance of each microbial species can then be calculated by multiplying the relative abundance by the total microbial quantity.
Example:
If qPCR reveals that the total bacterial count in a sample is 1 million, and the relative abundance of species A is 20%, the absolute abundance of species A would be:

Figure 2. The formula for calculating absolute abundance
Summary
In 16S sequencing, relative abundance is the primary method of analysis because it eliminates the variability caused by differences in sequencing depth. If absolute abundance is required, quantitative techniques must be incorporated to supplement the data.
Absolute and Relative Abundance in Metagenomic Sequencing
Metagenomic sequencing involves directly sequencing the genomes of all microorganisms present in a sample, providing a more comprehensive analysis. This method allows for the detection of bacteria, fungi, viruses, and other microbial genetic information. Metagenomic sequencing offers higher resolution and enables direct insights into microbial functional characteristics.
Similar to 16S rRNA sequencing, metagenomic sequencing typically utilizes relative abundance for data analysis. This is due to the fact that the total number of sequence reads in metagenomic sequencing can be influenced by sequencing depth, leading to significant variation in the total read count between samples. Therefore, the use of relative abundance ensures comparability across samples.
Absolute Abundance in Metagenomics
To determine the absolute abundance of each microbial species in metagenomic sequencing, total microbial abundance data for the sample is required. As with 16S rRNA sequencing, methods such as qPCR or other quantitative techniques can be employed to estimate total microbial load. The absolute abundance for each microorganism can then be calculated by multiplying the relative abundance by the estimated total abundance.
Metagenomics vs. 16S rRNA Sequencing: Abundance Metrics
16S rRNA Sequencing: This method is more suitable for quickly assessing the composition and changes in microbial communities, especially when budget constraints exist. The calculation of relative abundance is straightforward; however, the resolution is limited due to the sequencing of only a specific fragment of the 16S gene, making it challenging to obtain accurate absolute counts.
Metagenomic Sequencing: This approach captures a broader range of microbial taxa, including bacteria, viruses, fungi, and other organisms, while also providing more in-depth analysis of microbial functional traits. Although metagenomic sequencing is more costly, it offers richer information, including gene functions and ecological roles. Like 16S rRNA sequencing, metagenomic sequencing primarily relies on relative abundance analysis unless supplemented with quantitative techniques to derive absolute abundance.
Advantages of CD Genomics' Amplicon Absolute Quantification Sequencing Technology
Comprehensive Data Acquisition: The technology enables the simultaneous generation of three distinct datasets from a single test, thereby providing a richer and more detailed result.
Minimized Sample Requirements: The method requires a lower sample volume, reducing the risk of data loss due to insufficient sample availability or the absence of backups.
High Throughput and Sensitivity: The technology offers high throughput, with the ability to achieve absolute quantification for a wide range of microbial species within the detection range, ensuring comprehensive analysis.
Complementary Relative and Absolute Quantification: The combination of relative and absolute quantification provides a robust validation of results, reducing the occurrence of false positives commonly associated with traditional relative quantification methods.
Elimination of Cross-Platform qPCR Systematic Errors: The approach mitigates systematic biases often encountered in cross-platform qPCR quantification, ensuring more reliable results.
Superior Specificity and Sensitivity: The internal standard method offers higher specificity, sensitivity, and reproducibility in quantification compared to conventional qPCR techniques.
Minimized PCR Inhibition: The method reduces the impact of residual PCR inhibitors from DNA extraction processes, ensuring the accuracy and reliability of the results.
Simplified Primer Design and Optimization: The approach avoids the challenges typically encountered in primer design and optimization that are inherent to qPCR and other quantitative assays.
Summary
Absolute and relative abundance are two essential concepts in microbiome research. Absolute abundance provides the actual count of microorganisms in a sample, while relative abundance describes the proportional representation of each microorganism within the sample.
In the context of 16S rRNA and metagenomic sequencing, both types of abundance have specific applications:
- 16S rRNA Sequencing: Relative abundance is primarily used for community structure analysis. If absolute abundance is needed, it must be determined through supplementary techniques, such as qPCR, to quantify the total microbial load.
- Metagenomic Sequencing: While relative abundance is used to gain a comprehensive understanding of microbial communities and their functions, absolute abundance requires quantitative methods to estimate total microbial abundance.
This article aims to clarify the abundance-related concepts in these sequencing technologies and provide guidance on how to apply both absolute and relative abundance effectively in microbiome studies.